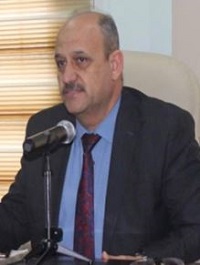
Geometric Function Theory in Real Life Applications
Geometric Function Theory in Real Life Applications
Prof. Dr. Abdul-Rahman S. Juma-Department of Mathematics
eps.abdulrahman.juma@uoanbar.edu.iq
Geometric Function Theory
Geometric Function Theory is the branch of Complex Analysis which deals with the geometric properties of analytic functions. The famous Riemann mapping theorem about the replacement of an arbitrary domain (of analytic function) with the open unit disk is the founding stone of the geometric function theory. Later, Koebe (1907) and Bieberbach (1916) studied analytic univalent functions which map E onto the domain with some nice geometric properties. Such functions and their generalizations serve a key role in applications, such as; Image processing, signal processing. Functions with bounded turning, that is, functions whose derivative has positive real part and their generalizations have very close connection to various classes of analytic univalent functions. These classes have been considered by many mathematicians such as Noshiro & Warchawski (1935), Chichra (1977), Goodman (1983) and Noor (2009).
A rectangular grid (top) and its image under a conformal map f (bottom). It is seen that f maps pairs of lines intersecting at 90° to pairs of curves still intersecting at 90°.
Some Applications of Geometric Function Theory
1- The results of geometric function theory are used in many areas of applied mathematics from mathematical physics to computer science. 2- However the previous work on this subject already found applications, sometimes unexpected, such as; control theory, Material science, Computer science, Signal processing, Mathematical physics, Astrophysics and Video game programming
Geometric Function Theory for Image Representation
1- Nowadays, in the digital era, the problems of analyzing and managing the information in big data, much of which is. 2- Visual, make the issue of more efficient image representations. 3- Image representations are important both for computational processing of image data, intermediate transformations and feature extraction as well as for higher-level cognitive tasks such as building symbolic descriptions and ultimate image understanding.
Image Sampling
Sampling and Quantization
What is an image?
We can think of an image as a function, f, from R2 to R: f( x, y ) gives the intensity at position ( x, y ). Realistically, we expect the image only to be defined over a rectangle, with a finite range: f: [a,b]x[c,d] à [0,1]. A color image is just three functions pasted together. We can write this as a “vector-valued” function..
Images as functions
What is a digital image?
We usually operate on digital (discrete) images: 1-Sample the 2D space on a regular grid. 2-Quantize each sample (round to nearest integer). If our samples are D apart, we can write this as: f[i ,j] = Quantize{ f(i D, j D) }. The image can now be represented as a matrix of integer values.
Image processing
1- An image processing operation typically defines a new image g in terms of an existing image f.
2- We can transform either the range of f. Or the domain of f:
What kinds of operations can each perform?
Images – Capturing and Processing
Capturing Real-World Images
Picture – two dimensional image captured from a real-world scene that represents a momentary event from the 3D spatial world
Image Concepts
1- An image is a function of intensity values over a 2D plane I(r,s). 2- Sample function at discrete intervals to represent an image in digital form: - Matrix of intensity values for each color plane, - Intensity typically represented with 8 bits. 3- Sample points are called pixels.
Digital Images
1- Samples = pixels, 2- Quantization = number of bits per pixel. 3- Example: if we would sample and quantize standard TV picture (525 lines) by using VGA (Video Graphics Array), video controller creates matrix 640x480pixels, and each pixel is represented by 8 bit integer (256 discrete gray levels)
Application to texture analysis
1- A task of particular interest in artificial vision is the characterization or description of textures. The problem here is to find interesting features to describe a given texture. In other words, if we can compute well describing features, we can better characterize a texture. 2- It begin by examining the use of monogenic representation for local characterization of a textured object depicted